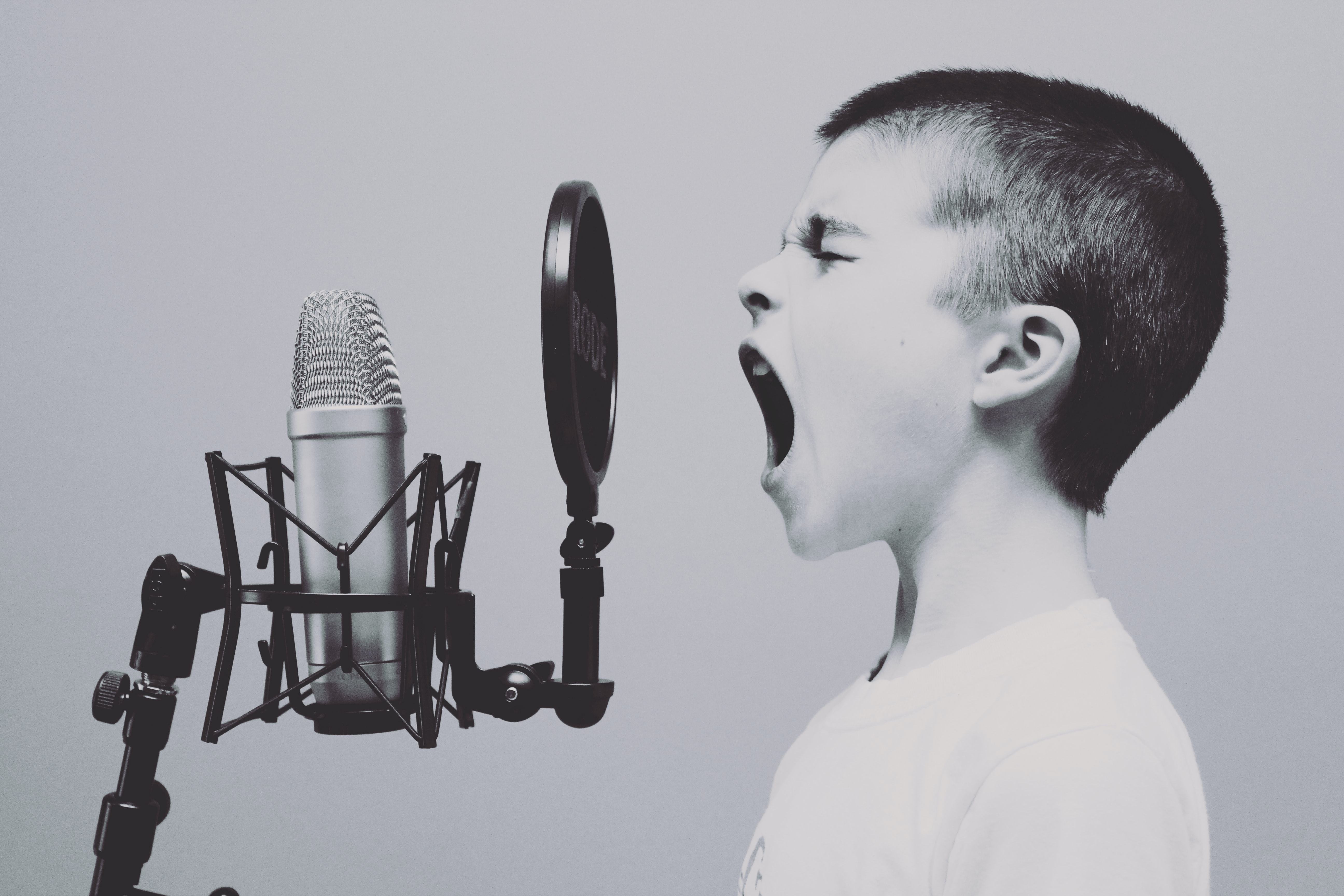
Importance of Good Incident Communication
From alerting to during to post incident, great communication is the key to effective incident response.
September 26, 2024
5 mins
AI is transforming how teams handle incidents. Designed to super power responders, AI tools can unlock reduced MTTRs and improved communication. Learn best practices when implementing AI strategies in your incident management process.
The first AI applications can be traced back to the ’60s and ’70s. They were called “expert systems” back then, but they would fall under the umbrella we call AI today. An early example was DENDRAL, used in the pharmaceutical industry to identify molecular structures. Before expert systems, scientists would take days or even weeks to identify a structure from a spectrometry sample. With DENDRAL, the task could be done in just a few hours.
DENDRAL was never meant to replace scientists, but to augment their capabilities through powerful tools. The current wave of AI focuses on the same: helping highly-skilled professionals get more done while sparing them repetitive and tedious tasks.
AI in incident response can be applied at several stages of the incident response process. Google is writing incident summaries 51% faster, Meta is reducing the root cause search from thousands of diffs to a handful, and Rootly AI users are seeing up to a 91% reduction in their MTTR thanks to AI assisting responders at every stage of the incident management process.
Incidents require responders to navigate a lot of complexity: endless traces to explore, hundreds of lines of code to check, and dozens of deployments to survey. As Steve McGhee, Reliability Advocate at Google, puts it: “you're building a system in your head as you're reading it, and you're comparing it to your history of what you just saw happen in real life.”
Today’s AI is still far from replacing a reliability engineer, but it can help them make better decisions faster and do a lot of menial work for them. From suggesting which teammates to invite to the response team to writing the retrospective, AI makes responders more effective.
{{cta-ai}}
Once an alert is acknowledged, the race towards resolution begins. Every minute counts, especially for high-severity incidents. That’s why Mean Time to Resolution (MTTR) has been a key reliability metric across stakeholders for decades—it signals how quickly you can recover and thus minimize financial and reputational damage.
By introducing AI at different stages of incident response, you can significantly reduce the time it takes to mitigate an incident. For example, teams using Rootly AI gain access to similar incidents for insights on what worked or was tried in the past. Or they can get suggestions on who helped resolve similar incidents before, expediting the incident response team formation.
Large organizations are exploring more sophisticated ways to reduce their MTTR with AI. Meta implemented a Root Cause Analyzer with a 43% accuracy rate. The system uses seven layers of heuristic filters to narrow down thousands of code changes to five potential culprits, allowing responders to evaluate problems more quickly.
Incidents often mobilize the entire organization, not just engineers. Executives, account managers, customer support representatives, PR specialists, and other incident response team roles may need to be kept in the loop. Unfortunately, this means that responders must couple their investigation and mitigation processes with answering queries and handling bureaucratic requirements.
GenAI can use the incident context to help write summaries about the situation, its impact, and what is being done to mitigate it. Rootly AI uses your incident’s context to draft summaries for stakeholders or bring new responders up to speed by responding to queries like, @rootly tell me what's been tried so far
.
Google has seen their responders save up to 51% of the time writing summaries by using GenAI. Their security team implemented an LLM that reads incident communications and drafts a summary, which must be approved by a human to ensure quality and accuracy.
Incidents require everyone involved to move as quickly as possible while dealing with difficult problems. Human error is common when logging incident events or keeping track of tasks that need to be done.
Incident management processes can leverage AI to log and construct accurate timelines of how an incident response developed and who did what. It can also suggest missing action items based on the playbooks defined by the organization.
Large language models (LLMs) have rapidly advanced in sophistication in recent years. This kind of AI can be trained to understand an incident’s context and pick up relevant events in the midst of the noise that incident coordination typically causes.
You can use AI to draft summaries of the incident situation and key developments to ensure your incident’s description stays up to date without requiring too much time. The same mechanism can be used to write a resolution message when the time comes.
Another idea is training an AI agent, like Rootly AI Copilot, that is available in your Slack channel to answer any questions about the incident. Newcomers can ask the AI to bring them up to speed, or responders can request the AI to draft a summary for executives.
Most SRE teams use workflows to automate tasks when dealing with incidents. Common examples include sending notifications to leadership when a new incident reaches a SEV2 severity level or syncing incident data with its Jira ticket counterpart.
You can use AI as part of your workflows to take more work off responders' hands. For example, you can plug in AI summarization into a workflow to ensure executives get timely updates on a high-severity incident every 20 minutes. Or, have AI track tasks to be completed post-incident (e.g., “we should look into periodic key rotations for XYZ service”) and automatically register them in Jira so they aren’t forgotten.
Rootly lets you integrate AI into your workflows, which also support native integrations with over 70+ tools that your team already uses.
Running a good retrospective, or incident postmortem, can help your team turn failures into learning opportunities. However, after an incident is resolved, the team has likely had more than enough to deal with. Make retrospectives feel less like a burden and more like actionable insights by simplifying the process with AI.
You can use your incident context-aware AI to prepare most of the materials you need for a retrospective meeting. Have the AI review the incident history to extract key points, affected systems, what was attempted, and how the incident was ultimately resolved.
Rootly offers a comprehensive suite for easier retrospectives, which can be enhanced with AI features like summarization and action item suggestions.
Implementing an AI solution for your SRE team will likely involve using agents provided by OpenAI or Wastonx. That means you’ll be transferring data, potentially including sensitive information such as PII, to an external processor. You need to ensure your data safety applies to AI and that your provider is not using it to train models that could end up helping your competition.
At Rootly, we use a privacy-first AI agent powered by Enterprise OpenAI. Rootly strips out any sensitive information before sending it to OpenAI for processing. We ensure your data is never stored or used for training purposes. You can also connect your own OpenAI account to maintain tighter control over data flow.
Rootly AI is like having your most experienced engineer in every incident. It guides you with helpful tips and steps using context from past incidents, so you're never left guessing what to do next. It automates tasks best left to machines, allowing you to focus on what's most important. Book a demo with our team today to explore Rootly's advanced AI features:
Utilize AI for configuration, finding, substitutions, generating reports, and more.